Statistical Modeling for Forecasting Fertilizer Consumption in India
DOI:
https://doi.org/10.14719/pst.1982Keywords:
ACF, ARIMA, Fertilizer consumption, Forecasting, Model selection criteria, PACF, Residual AnalysisAbstract
Fertilizers have contributed significantly to increased agricultural yields, particularly for cereal crops and they will still be an important part of the science-based farming that is needed to feed the world's growing population. Fertilizers replenish the soil nutrients lost by the harvested crops, promote the use of high-yielding cultivars and boost biomass in tropical soils that are deficient in nutrients. In this study, data on fertilizer consumption in India was gathered from Agricultural Statistics at a Glance from 1950-51 to 2020-21 and utilized to fit the ARIMA model and forecast future usage. Forecasting has been done using the Box-Jenkins ARIMA approach. The ARIMA model is the most popular and widely applied forecasting model for time series data. The data was calculated using autocorrelation and partial autocorrelation functions. R programming software was used to estimate model parameters. The performance of the fitted model was evaluated using various goodness of fit criteria, such as AIC, BIC and MAPE. Empirical results revealed that the ARIMA (1,2,1) model was best suited to forecasting India's future total fertilizer use. Similarly, the ARIMA model was fitted for nitrogen, phosphorus, and potassium consumption in India independently. Forecasts from 2021-22 to 2030-31 are calculated using the chosen model. By 2030-31, total fertilizer use is predicted to reach 32,058.55 thousand tonnes. Policymakers should preferably base their judgments on reliable forecasts in order to tighten policies and achieve outcomes. Predicting future events using an appropriate time series model will assist policymakers, marketing strategies in making decisions related to export/ import and developing appropriate fertilizer consumption strategies.
Downloads
References
FAO. World Agriculture towards 2030/2050: The 2012 Revision; 2012. https://www.fao.org/3/ap106e/ap106e.pdf
Smil V. Nitrogen and food production: Proteins for human diets. Ambio; (cited in FAO, 2006); 2002;31:126-13. https://doi.org/10.1579/0044-7447-31.2.126
Tomich T, Kilby P, Johnson, B. Transforming Agrarian Economies: Opportunities Seized, Opportunities Missed. Ithaca, NY: Cornell University Press; 1995. https://doi.org/10.7591/9781501717499
Hopper W. Indian Agriculture and Fertilizer: An Outsider’s Observations. Keynote address to the FAI Seminar on Emerging Scenario in Fertilizer and Agriculture: Global Dimensions, New Delhi, India; 1993.
FAO (Food and Agriculture Organization of the UN). Guide to Efficient Plant Nutrition Management. Rome, Italy; 1998.
Bumb B. Global Fertilizer Perspective, 1980–2000: The Challenges in Structural Transformation. Technical Bulletin T-42. Muscle Shoals, AL: International Fertilizer Development Center; 1995.
Box GEP, Jenkins GM. Time series Analysis, Forecasting and Control. San Francisco, Holden Day, California, USA; 1976.
Brown RG. Statistical Forecasting for Inventory Control. McGraw Hill Book Co., Inc., NY, USA; 1959.
Ljung GM, Box GEP. On a measure of lack of fit in time series models. Biometrika. 1978; 65:67-72. https://doi.org/10.1093/biomet/65.2.297
Pindyck RS, Rubinfeld DL. Econometric Models and Economic Forecasts. McGraw Hill Book Co. Inc., NY, USA;1981.
Badmus MA, Ariyo OS. Forecasting cultivated areas and production of maize in Nigeria using ARIMA model. Asian J Agric Sci. 2011;3(3):171-76.
Falak S, Eatzaz A. Forecasting Wheat production in Pakistan. Lahore J Econ. 2008;3(1):57-85. https://doi.org/10.35536/lje.2008.v13.i1.a3
Muhammed F, Siddique M, Bashir M, Ahamed S. Forecasting rice production in Pakistan using ARIMA models. J Anim Plant Sci. 1992;2:27-31.
Shabur SA, Haque ME. Analysis of rice in Mymensingh town market pattern and forecasting. Bang J Agric Econ. 1993;16:130-33.
Sohail A, Sarwar A, Kamran M. Forecasting total food grains in Pakistan. J Eng Appl Sci. 1994;13:140-46.
Slutsky E. The Summation of Random Causes as a Source of Cyclic Processes. Problems of Economic Conditions, 1927;3(1):
Wold HOA. A Study of the Analysis of Stationary Time Series. (2nd ed. 1954). Uppsala: Almqvist and Wiksells. 1938.
Yule GU. Why do we sometimes get nonsense-correlations between time-series? A study in sampling and the nature of time-series. Journal of the Royal Statistical Society.1926;89(1): 1-63. https://doi.org/10.2307/2341482
Kathayat B, Dixit AK. Paddy price forecasting in India using ARIMA model. Journal of Crop and Weed. 2021;17(1):48-55. https://doi.org/10.22271/09746315.2021.v17.i1.1405
Mishra P, Yonar A, Yonar H, Binita K, Abotaleb M. State of the art in total pulse production in major states of India using ARIMA techniques. Current Research in Food Science. 2021;4:800-06. https://doi.org/10.1016/j.crfs.2021.10.009
Mao L, Huang Y, Zhang X, Li S, Huang X. ARIMA model forecasting analysis of the prices of multiple vegetables under the impact of the COVID-19. PLos ONE.2022;17(7):1-26. https://doi.org/10.1371/journal.pone.0271594
Kannan S, Karuppasamy KM. Forecasting for agricultural production usisng ARIMA model. PalArch’s Journal of Archaeology of Egypt/Egyptology. 2020;17(9):5939-49.
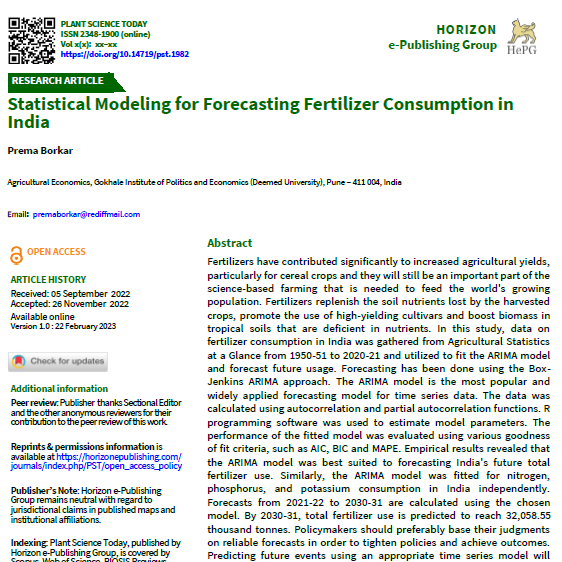
Downloads
Published
Versions
- 01-04-2023 (2)
- 22-02-2023 (1)
How to Cite
Issue
Section
License
Copyright (c) 2022 Prema Borkar

This work is licensed under a Creative Commons Attribution 4.0 International License.
Copyright and Licence details of published articles
Authors who publish with this journal agree to the following terms:
- Authors retain copyright and grant the journal right of first publication with the work simultaneously licensed under a Creative Commons Attribution License that allows others to share the work with an acknowledgement of the work's authorship and initial publication in this journal.
- Authors are able to enter into separate, additional contractual arrangements for the non-exclusive distribution of the journal's published version of the work (e.g., post it to an institutional repository or publish it in a book), with an acknowledgement of its initial publication in this journal.
Open Access Policy
Plant Science Today is an open access journal. There is no registration required to read any article. All published articles are distributed under the terms of the Creative Commons Attribution License (CC Attribution 4.0), which permits unrestricted use, distribution, and reproduction in any medium, provided the original author and source are credited (https://creativecommons.org/licenses/by/4.0/). Authors are permitted and encouraged to post their work online (e.g., in institutional repositories or on their website) prior to and during the submission process, as it can lead to productive exchanges, as well as earlier and greater citation of published work (See The Effect of Open Access).