Field-level rice yield estimations under different farm practices using the crop simulation model for better yield
DOI:
https://doi.org/10.14719/pst.2690Keywords:
Crop model, DSSAT, rice, sowing, LAIAbstract
Crop yield estimation is essential for decision-making systems and insurance policy makers. Numerous methodologies for yield estimation have been developed, encompassing crop models, remote sensing techniques, and empirical equations. Each approach holds unique limitations and advantages. The primary aim of this study was to assess the accuracy of the DSSAT (Decision Support System for Agro Technology Transfer) model in predicting rice yields and LAI (Leaf Area Index) across various management methods. Additionally, the study sought to identify the optimal management practice for attaining higher yields. Crop models facilitate the expeditious evaluation of management strategies aimed at improving crop yield and analyzing the balance between production, resource efficiency, and environmental impacts. The study region selected for analysis is Karimnagar district of Telangana state. DSSAT has been chosen as the preferred tool due to its high efficiency in evaluating crop yield. The model's simulated yield was compared to the observed yield obtained from crop-cutting experiments. The results indicate a correlation of 0.81 and 0.85 between observed and simulated yields, as well as between model LAI and yield. An observation was made regarding a discrepancy between predicted and actual yields, which can be attributed to biotic stress. However, it should be noted that the current model does not account for this factor. The observed average yield was 5200 kg ha-1, whereas the projected yield was 5400 kg ha-1. The findings indicate that the model's performance is influenced by both the timing of sowing and the amount of nitrogen applied. The findings indicate that the DSSAT model has demonstrated a high level of accuracy in predicting both yields and leaf area index (LAI) across various management strategies. This study showcases the potential use of crop simulation models as a technology-driven tool to identify the most effective management strategies for rice production.
Downloads
References
Agriculture USDo. FY 2021 Performance report. 2021.
Akinbile CO, Sangodoyin AY. Evapotranspiration, soil and water quality implications on upland rice production. Asian Journal of Crop Science. 2011;3(4):169-78.
Government of Telangana. Season and crop covergae report vanakalam-2021," report2021. 2021.
Jin X, Li Z, Yang G, Yang H, Feng H, Xu X, et al. Winter wheat yield estimation based on multi-source medium resolution optical and radar imaging data and the AquaCrop model using the particle swarm optimization algorithm. ISPRS Journal of Photogrammetry and Remote Sensing. 2017;126:24-37.
Basso B, Gargiulo O, Paustian K, Robertson GP, Porter C, Grace PR, et al. Procedures for initializing soil organic carbon pools in the DSSAT?CENTURY model for agricultural systems. Soil Science Society of America Journal. 2011;75(1):69-78.
Zhang D, Wang H, Li D, Li H, Ju H, Li R, et al. DSSAT-CERES-Wheat model to optimize plant density and nitrogen best management practices. Nutrient Cycling in Agroecosystems. 2019;114:19-32.
Ma G, Huang J, Wu W, Fan J, Zou J, Wu S. Assimilation of MODIS-LAI into the WOFOST model for forecasting regional winter wheat yield. Mathematical and Computer Modelling. 2013;58(3-4):634-43.
Jones JW, Hoogenboom G, Porter CH, Boote KJ, Batchelor WD, Hunt L, et al. The DSSAT cropping system model. European journal of agronomy. 2003;18(3-4):235-65.
He J, Dukes MD, Hochmuth GJ, Jones JW, Graham WD. Identifying irrigation and nitrogen best management practices for sweet corn production on sandy soils using CERES-Maize model. Agricultural Water Management. 2012;109:61-70.
Amarasingha R, Suriyagoda L, Marambe B, Rathnayake W, Gaydon D, Galagedara L, et al. Improving water productivity in moisture-limited rice-based cropping systems through incorporation of maize and mungbean: A modelling approach. Agricultural water management. 2017;189:111-22.
Cooper M, van Eeuwijk FA, Hammer GL, Podlich DW, Messina C. Modeling QTL for complex traits: detection and context for plant breeding. Current opinion in plant biology. 2009;12(2):231-40.
Trienekens J, Top J, van der Vorst J, Beulens A. Towards effective food chains: models and applications: Wageningen Academic Publishers; 2010.
Angulo C, Becker M, Wassmann R. Yield gap analysis and assessment of climate-induced yield trends of irrigated rice in selected provinces of the Philippines. Journal of Agriculture and Rural Development in the Tropics and Subtropics (JARTS). 2012;113(1):61-8.
Grassini P, van Bussel LG, Van Wart J, Wolf J, Claessens L, Yang H, et al. How good is good enough? Data requirements for reliable crop yield simulations and yield-gap analysis. Field Crops Research. 2015;177:49-63.
Yang K-W, Chapman S, Carpenter N, Hammer G, McLean G, Zheng B, et al. Integrating crop growth models with remote sensing for predicting biomass yield of sorghum. in silico Plants. 2021;3(1):diab001.
Roja M, Kumar K, Ramulu V, Deepthi C. Modeling and evaluation of aquacrop for maize (Zea mays L.) under full and deficit irrigation in semi-arid tropics. Indian Journal of Agricultural Research. 2021;55(4):428-33.
Gumma MK, Kadiyala M, Panjala P, Ray SS, Akuraju VR, Dubey S, et al. Assimilation of remote sensing data into crop growth model for yield estimation: A case study from India. Journal of the Indian Society of Remote Sensing. 2022;50(2):257-70.
Kadiyala M, Jones J, Mylavarapu R, Li Y, Reddy M. Identifying irrigation and nitrogen best management practices for aerobic rice–maize cropping system for semi-arid tropics using CERES-rice and maize models. Agricultural Water Management. 2015;149:23-32.
Parker GG. Tamm review: Leaf Area Index (LAI) is both a determinant and a consequence of important processes in vegetation canopies. Forest Ecology and Management. 2020;477:118496.
Yan G, Hu R, Luo J, Weiss M, Jiang H, Mu X, et al. Review of indirect optical measurements of leaf area index: Recent advances, challenges, and perspectives. Agricultural and forest meteorology. 2019;265:390-411.
Asner GP, Scurlock JM, A. Hicke J. Global synthesis of leaf area index observations: implications for ecological and remote sensing studies. Global Ecology and Biogeography. 2003;12(3):191-205.
Ren H, Liu R, Yan G, Mu X, Li Z-L, Nerry F, et al. Angular normalization of land surface temperature and emissivity using multiangular middle and thermal infrared data. IEEE Transactions on Geoscience and Remote Sensing. 2014;52(8):4913-31.
Stark SC, Leitold V, Wu JL, Hunter MO, de Castilho CV, Costa FR, et al. Amazon forest carbon dynamics predicted by profiles of canopy leaf area and light environment. Ecology letters. 2012;15(12):1406-14.
Hui J, Yao L. A Method to Upscale the Leaf Area Index (LAI) Using GF-1 Data with the Assistance of MODIS Products in the Poyang Lake Watershed. Journal of the Indian Society of Remote Sensing. 2018;46(4):551-60.
Yu Y, Wang J, Liu G, Cheng F. Forest Leaf Area Index Inversion Based on Landsat OLI Data in the Shangri-La City. Journal of the Indian Society of Remote Sensing. 2019;47(6):967-76.
Hoogenboom G, Jones J, Wilkens P, Porter C, Boote K, Hunt L, et al. Decision support system for agrotechnology transfer (DSSAT) Version 4.5 [CD-ROM]. University of Hawaii, Honolulu, Hawaii. 2010.
Liu H, Yang J, Drury Ca, Reynolds W, Tan C, Bai Y, et al. Using the DSSAT-CERES-Maize model to simulate crop yield and nitrogen cycling in fields under long-term continuous maize production. Nutrient cycling in agroecosystems. 2011;89:313-28.
Salmerón M, Urrego Y, Isla R, Cavero J. Effect of non-uniform sprinkler irrigation and plant density on simulated maize yield. Agricultural water management. 2012;113:1-9.
Jeong H, Jang T, Seong C, Park S. Assessing nitrogen fertilizer rates and split applications using the DSSAT model for rice irrigated with urban wastewater. Agricultural water management. 2014;141:1-9.
Corbeels M, Chirat G, Messad S, Thierfelder C. Performance and sensitivity of the DSSAT crop growth model in simulating maize yield under conservation agriculture. European journal of agronomy. 2016;76:41-53.
Si Z, Zain M, Li S, Liu J, Liang Y, Gao Y, et al. Optimizing nitrogen application for drip-irrigated winter wheat using the DSSAT-CERES-Wheat model. Agricultural Water Management. 2021;244:106592.
Hargreaves GH, Samani ZA. Reference crop evapotranspiration from temperature. Applied engineering in agriculture. 1985;1(2):96-9.
Batjes N. ISRIC-WISE global data set of derived soil properties on a 0.5 by 0.5 degree grid (Version 3.0). ISRIC-World Soil Information, Wageningen. 2005.
Willmott CJ, Ackleson SG, Davis RE, Feddema JJ, Klink KM, Legates DR, et al. Statistics for the evaluation and comparison of models. Journal of Geophysical Research: Oceans. 1985;90(C5):8995-9005.
Garnier P, Neel C, Mary B, Lafolie F. Evaluation of a nitrogen transport and transformation model in a bare soil. European journal of soil science. 2001;52(2):253-68.
Ahmad S, Ahmad A, Soler CMT, Ali H, Zia-Ul-Haq M, Anothai J, et al. Application of the CSM-CERES-Rice model for evaluation of plant density and nitrogen management of fine transplanted rice for an irrigated semiarid environment. Precision Agriculture. 2012;13:200-18.
Yarnia M. Evaluation of CERES-Rice Model in Simulation of Rice Growth under Constraint Irrigation and Nitrogen Fertilizer Conditions. 2016.
Shibu ME, Leffelaar PA, Van Keulen H, Aggarwal PK. LINTUL3, a simulation model for nitrogen-limited situations: Application to rice. European Journal of Agronomy. 2010;32(4):255-71.
Lampayan R, Bouman B, De Dios J, Espiritu A, Soriano J, Lactaoen A, et al. Yield of aerobic rice in rainfed lowlands of the Philippines as affected by nitrogen management and row spacing. Field crops research. 2010;116(1-2):165-74.
Fageria N. Yield physiology of rice. Journal of plant nutrition. 2007;30(6):843-79.
Abayechaw D. Review on Decision Support System for Agrotechnology Transfer (DSSAT) Model. Agrotechnology. 2021;10(11):236.
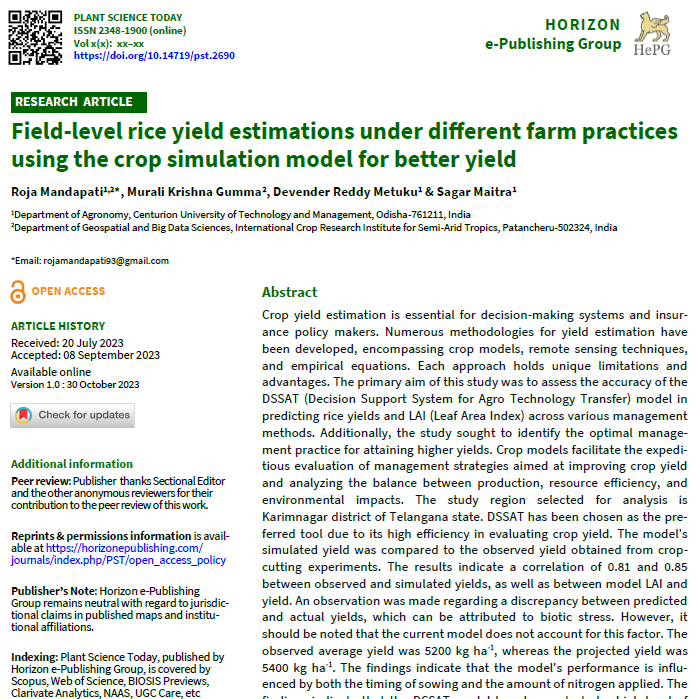
Downloads
Published
Versions
- 04-01-2024 (2)
- 30-10-2023 (1)
How to Cite
Issue
Section
License
Copyright (c) 2022 Roja Mandapati, Murali Krishna Gumma, Devender Reddy Metuku, Sagar Maitra

This work is licensed under a Creative Commons Attribution 4.0 International License.
Copyright and Licence details of published articles
Authors who publish with this journal agree to the following terms:
- Authors retain copyright and grant the journal right of first publication with the work simultaneously licensed under a Creative Commons Attribution License that allows others to share the work with an acknowledgement of the work's authorship and initial publication in this journal.
- Authors are able to enter into separate, additional contractual arrangements for the non-exclusive distribution of the journal's published version of the work (e.g., post it to an institutional repository or publish it in a book), with an acknowledgement of its initial publication in this journal.
Open Access Policy
Plant Science Today is an open access journal. There is no registration required to read any article. All published articles are distributed under the terms of the Creative Commons Attribution License (CC Attribution 4.0), which permits unrestricted use, distribution, and reproduction in any medium, provided the original author and source are credited (https://creativecommons.org/licenses/by/4.0/). Authors are permitted and encouraged to post their work online (e.g., in institutional repositories or on their website) prior to and during the submission process, as it can lead to productive exchanges, as well as earlier and greater citation of published work (See The Effect of Open Access).