Linear mathematical models for yield estimation of baby corn (Zea mays L.)
DOI:
https://doi.org/10.14719/pst.2618Keywords:
Baby corn, crop yield estimation, maize, mathematical modeling, regression-based models, yield predictionAbstract
Linear mathematical models have been developed for predicting baby corn yield in terms of cob volume for two cycles of maize (Zea mays L.). Cob volume is directly proportional to morphological parameters such as length, weight, and girth; hence, linear mathematical models have been developed. Primary data for a random selection of 60 cobs for each cycle were collected, and lab work was carried out to measure the corn ears and cob growth parameters. An irregular distribution was observed among all six growth parameters examined in the study. Descriptive statistical measures were employed to facilitate the description of growth parameters. The final volume of the baby corn cob was used for crop yield estimation. The water displacement method was employed to measure the actual volume of cobs, which was then compared with the volumes estimated using the developed mathematical models. For both cycles, similar trends were observed in both estimated and actual volumes of cobs, providing numerical confirmation for the validity of the developed mathematical models. The theoretical validity of these models was also established using statistical measures such as R2, adjusted R2, F-test, P-value, and correlation coefficient. Any deviations between estimated and actual volumes would indicate changes in the dependent variables of the model, attributed to the effects of climate change, as other internal and external factors are held constant. These models offer a critical predictive tool for stakeholders, enabling improved yield predictions and optimized resource allocation. As a result, they facilitate strategic planning for increased profitability.
Downloads
References
Solomon S, Qin D, Manning M,Chen Z, Marquis M, Averyt KB, Tignor M, Miller HL, editors. Climate change 2007: The physical science basis. Contribution of working group I to the fourth assessment report of the intergovernmental panel on climate change. Cambridge University Press, Cambridge, United Kingdom and New York, NY, USA.2007;p.1-10.
Challinor AJ, Watson J, Lobell DB, Howden SM, Smith DR, Chhetri N. A meta-analysis of crop yield under climate change and adaptation. Nat Clim Change. 2014;4:287-91.https://doi.org/10.1038/nclimate2153
Liu Y, Li N, Zhang Z, Huang C, Chen X, Wang F. The central trend in crop yields under climate change in China: A systematic review. Sci of the Total Environ. 2020;704:1353-55. https://doi.org/10.1016/j.scitotenv.2019.135355
Talwar S, Tayal P, Kumar S, Bamel K, Prabhavathi V. Climate change: A threat to biodiversity. In: Proceedings of National Conference on “Climate Change: Impacts, Adaptation, Mitigation Scenario and Future challenges in Indian Perspective”. 2015;p. 84-93.
Talwar S, Bamel K, Prabhavathi. Impact of elevated temperature and carbon dioxide on seed physiology and yield. Plant Science Today. 2022a;9(sp):85-91. https://doi.org/10.14719/pst.2059
Talwar S, Bamel K, Prabhavathi, Mal A. Effect of high temperature on reproductive phases of plants- A review’. Nat Env Poll Tech. 2022b;21(4):1887-92. https://doi.org/10.46488/NEPT.2022.v21i04.043
Wing IS, De Cian E, Mistry MN. Global vulnerability of crop yields to climate change. J Environmental Economics and Management. 2021;109:1024-62. https://doi.org/10.1016/j.jeem.2021.102462
Bamel K, Bamel JS, Rani N, Pathak SK, Gahlot S, Singh RN. Crop yield prediction using satellite remote sensing based methods. Int J of Botany Stud. 2022a;7:35-40. https://www.botanyjournals.com/assets/archives/2022/vol7issue2/7-1-125-326.pdf
Rani N, Bamel K, Shukla A, Singh N. Analysis of five mathematical models for crop yield prediction. South Asian J Exp Biol. 2022;12(1):46-54. https://doi.org/10.38150/sajeb.12(1).p46-54
Basso B, Liu L. Seasonal crop yield forecast: Methods, applications and accuracies. In: D.L. Sparks (eds) Advances in Agronomy, Academic Press. 2019;154:201-55. https://doi.org/10.1016/bs.agron.2018.11.002
Bamel K, Rani N, Bamel JS, Gahlot S, Singh RN, Pathak SK. Current approaches and future perspectives in methods for crop yield estimation. Bull Environ Pharmacol Life Sci. 2022b;1:243-47. https://bepls.com/special_issue(1)2022/37.pdf
Singh AK, Kumar R, Bohra JS, Kumawat N. Fodder yield, nutrient uptake and quality of baby corn (Zea mays L.) as influenced by NPKS and Zn fertilisation. Res Crops. 2015;16:243-49. http://dx.doi.org/10.5958/2348-7542.2015.00036.4
Aggarwal PK, Kalra N, Chander S, Pathak, H. Info Crop: a dynamic simulation model for the assessment of crop yields, losses due to pests and environmental impact of agro-ecosystems in tropical environments. I. Model description. Agric Syst. 2006;89:1-25.https://doi.org/10.1016/j.agsy.2005.08.001
Bozokalfa MK, Kilic M. Mathematical modelling in the estimation of pepper (Capsicum annuum L.) fruit volume. Chilean J Agricultural Res. 2010;70:626-32.https://doi.org/10.4067/S0718-58392010000400013
Bresler E, Dagan G. Variability of yield of an irrigated crop and its causes: 1. Statement of the problem and methodology. Water Resour Res. 1988;24:381-87. https://doi.org/10.1029/WR024i003p00381
Hochmuth GJ, Cantliffe DJ, Soundy P. A comparison of three mathematical models of response to applied nitrogen: A case study using lettuce. Hort Sci. 1998;33:5.https://doi.org/10.21273/HORTSCI.33.5.833
Kropff MJ. Modelling the effects of weeds on crop production. Weed Res. 1998;28:465-71.https://doi.org/10.1111/j.1365-3180.1988.tb00829.x
Madadgar S, Kouchak A, Farahmand A, Davis SJ. Probabilistic estimates of drought impacts on agricultural production. Geophys Res Lett. 2017;44:7799-7807. https://doi.org/10.1002/2017GL073606
Magaji AS, Nasir MO. A discrete-time mathematical model for the control of weeds population density towards improving crop yields. Sci World J. 2019;14:74-77.
Osama S, Elkholy M, Kansoh RM. Optimization of the cropping pattern in Egypt. Alex Eng J. 2017;56:557-66.https://doi.org/10.1016/j.aej.2017.04.015
Overmars KP, de Groot WT, Huigen MG. Comparing inductive and deductive modeling of land use decisions: Principles, a model and an illustration from the Philippines. Human Ecol. 2007;35:439-52.https://doi.org/10.1007/s10745-006-9101-6
Green JR, Margerison D. Statistical treatment of experimental data. Elsevier Scientific Publishing Company, New York, USA; 1978.
Spiegel MR, Stephens LJ. Schaum’s Outline of Statistics, 3rd ed., McGraw-Hill, Columbus, Ohio, USA; 1998.
Sadenova MA, Beisekenov NA, Rakhymberdina MY, Varbanov PS, Klemes JJ. Mathematical modelling in crop production to predict crop yields. Chem Eng Transac. 2021;88:1225-30. https://doi.org/10.3303/CET2188204
Kumar P. Crop yield forecasting by adaptive neuro fuzzy inference system. Math TheoryMod. 2011;1:1-7.
Maroušek J, Minofar B, Maroušková A, Strunecký O, Gavurová B. Environmental and economic advantages of production and application of digestate biochar. Environ TechInnov. 2023;30:103-09. https://doi.org/10.1016/j.eti.2023.103109
Maroušek J, Trakal L. Techno-economic analysis reveals the untapped potential of wood biochar. Chemos. 2022;291:133000. https://doi.org/10.1016/j.chemosphere.2021.133000
Maroušek J, Maroušková A, Periakaruppan R, Gokul GM, Anbukumaran A, Bohatá A, K?íž P, Bárta J, ?erný P, Olšan P. Silica nanoparticles from coir pith synthesized by acidic sol-gel method improve germination economics. Polymers. 2022;14(2):266. https://doi.org/10.3390/polym14020266
Durana P, Perkins N, Valaskova K. Artificial intelligence data-driven internet of things systems, real-time advanced analytics and cyber-physical production networks in sustainable smart manufacturing. Econ Manag Financ Mark. 2021;16:20-30. https://doi.org/10.22381/emfm16120212
Zvarikova K, Rowland M, Krulicky T. Sustainable industry 4.0 wireless networks, smart factory performance and cognitive automation in cyber-physical system-based manufacturing. J Self-Govern Manag Econ. 2021;9(4). https://doi.org/10.22381/jsme9420211
Kovacova M, L?z?roiu G. Sustainable organizational performance, cyber-physical production networks and deep learning-assisted smart process planning in Industry 4.0-based manufacturing systems. Econ, Manag Finan Mar. 2021;16(3):41-54.
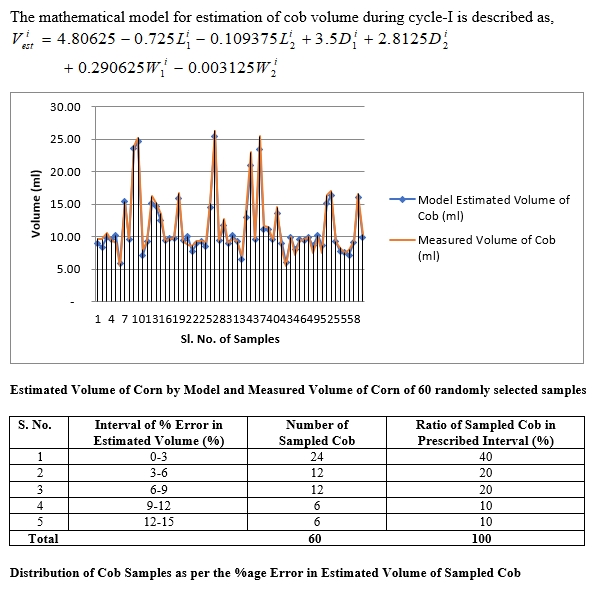
Downloads
Published
Versions
- 01-01-2024 (2)
- 16-10-2023 (1)
How to Cite
Issue
Section
License
Copyright (c) 2022 Neetu Rani, Jitender Singh Bamel, Savita Garg, Abhinav Shukla, Sumit Kumar Pathak, Rishta Nandini Singh, Nandini Singh, Sara Gahlot, Kiran Bamel

This work is licensed under a Creative Commons Attribution 4.0 International License.
Copyright and Licence details of published articles
Authors who publish with this journal agree to the following terms:
- Authors retain copyright and grant the journal right of first publication with the work simultaneously licensed under a Creative Commons Attribution License that allows others to share the work with an acknowledgement of the work's authorship and initial publication in this journal.
- Authors are able to enter into separate, additional contractual arrangements for the non-exclusive distribution of the journal's published version of the work (e.g., post it to an institutional repository or publish it in a book), with an acknowledgement of its initial publication in this journal.
Open Access Policy
Plant Science Today is an open access journal. There is no registration required to read any article. All published articles are distributed under the terms of the Creative Commons Attribution License (CC Attribution 4.0), which permits unrestricted use, distribution, and reproduction in any medium, provided the original author and source are credited (https://creativecommons.org/licenses/by/4.0/). Authors are permitted and encouraged to post their work online (e.g., in institutional repositories or on their website) prior to and during the submission process, as it can lead to productive exchanges, as well as earlier and greater citation of published work (See The Effect of Open Access).